Utilizing weather, soil, crop, and geographic data, agro-meteorological models improve agricultural sustainability and productivity. These models serve farmers by forecasting weather patterns, evaluating the effects of climate change, and streamlining resource management decisions related to irrigation, planting, harvesting, and pest control. They are essential for boosting crop yields, lowering risks, and encouraging resource efficiency, all of which support environmental sustainability and food security.
Table of Contents
Introduction to Agro-Meteorological Models
In order to increase farming’s productivity and efficiency, agro-meteorological models are advanced instruments that combine meteorological data with agricultural methods. These models act as a link between actual agricultural applications and the scientific understanding of weather patterns. Agro-meteorological models are able to forecast weather phenomena and evaluate their possible effects on crop growth and yield by utilizing both historical and current meteorological data.
These models’ basic idea is based on the relationship between agricultural outputs and meteorological factors like temperature, precipitation, humidity, and solar radiation. For example, soil moisture levels, pest and disease outbreaks, and crop development stages are all strongly influenced by temperature and rainfall patterns.
Types of Agro-Meteorological Models
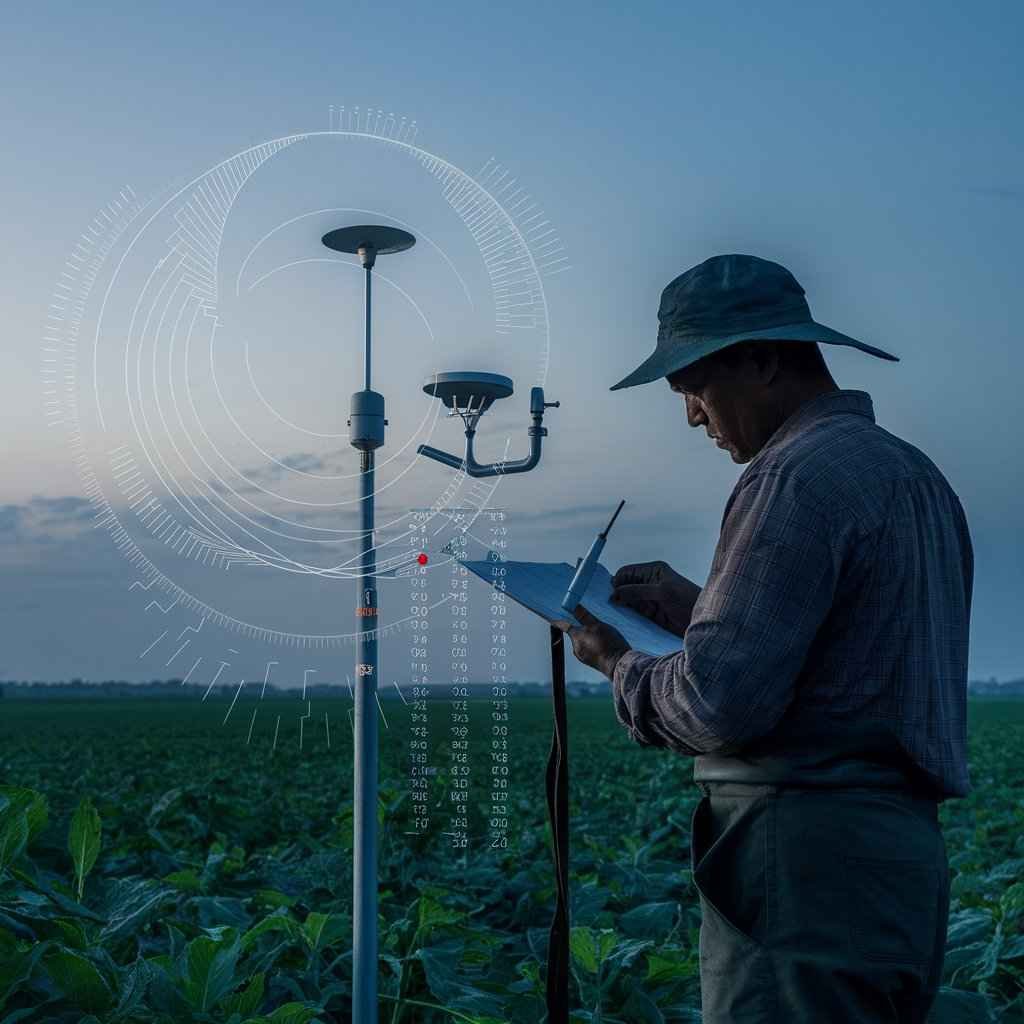
Agro-meteorological models are essential instruments in contemporary agriculture, helping to forecast and control a wide range of agricultural phenomena. Crop simulation models, pest and disease forecasting models, and climate impact models are the three main categories into which they can be divided. Every variety has a unique function and is designed to meet particular agricultural requirements.
1. Crop Growth Models
- Description: Replicate crop growth and development according to management techniques and environmental factors.
- Agrotechnology Transfer Decision Support System (DSSAT) and Agricultural Production Systems Simulator (APSIM) are two examples.
2. Weather Forecast Models:
- Description: Assist in scheduling agricultural activities by providing both short- and long-term weather predictions.
- The European Centre for Medium-Range Weather Forecasts (ECMWF) and the Weather Research and Forecasting Model (WRF) are two examples.
3. Climate Models:
- Description: Utilizing long-term climate scenarios, drive adaptation efforts by evaluating possible effects on agriculture.
- Two examples are Regional Climate Models (RCMs) and General Circulation Models (GCMs).
4. Pest and Disease Models:
- Description: Anticipate when diseases and pests may manifest and spread in response to different weather patterns so that prompt control measures can be put in place.
- CIPM (Integrated Pest Management models) and EPIRICE (a model for rice illnesses) are two examples.
5. Hydrological Models:
- Description: By modeling the flow and dispersal of water in agricultural landscapes, water resources can be evaluated and managed.
- Examples are WEAP (Water Evaluation and Planning System) and SWAT (Soil and Water Assessment Tool).
6. Soil Moisture Models:
- Description: To maximize irrigation and water management techniques, track and forecast soil moisture levels.
- AquaCrop and HYDRUS are two examples.
Applications and Benefits of Agro-Meteorological Models
Agro-meteorological models are essential to contemporary agriculture because they offer vital information that agronomists, farmers, and legislators may use to make wise decisions. These models use agricultural and meteorological data to forecast weather patterns, estimate crop yields, and improve the efficiency of agricultural resource management.
Crop management is one of the main uses cases for agro-meteorological models. These models help farmers arrange their planting and harvesting dates to avoid unfavorable situations by forecasting weather patterns. An agro-meteorological model, for instance, can help a farmer choose when to sow a crop in order to maximize production and reduce the chance of crop failure. Similarly, to guarantee that crops receive the proper quantity of resources at the right moment during the growth season, these models can assist in scheduling fertilization, irrigation, and pest control procedures.
Agro-meteorological models also provide considerable benefits in terms of resource optimization. These models assist farmers in making better use of water, fertilizers, and other inputs by offering precise weather forecasts. For example, a study in India found that utilizing agro-meteorological models to schedule irrigation lowered water consumption by 30% while maintaining or increasing crop production. This saves critical resources while simultaneously lowering production costs.
Agro-meteorological models can also help mitigate the effects of severe weather conditions. For example, in drought-prone areas, these models can forecast dry spells and assist farmers in taking preventive steps, such as planting drought-resistant crops or applying water-saving practices. In the case of catastrophic weather disasters such as hurricanes or frost, farmers can use accurate projections to protect their crops, animals, and infrastructure, reducing losses.
Challenges
Agro-meteorological models face several challenges that can impact their effectiveness and reliability. These challenges include:
1. Data Quality and Availability
- Accurate Data: Reliable model outputs require high-quality, high-resolution data, which can be challenging to get, particularly in rural or underdeveloped areas.
- Temporal and spatial coverage: Incomplete or inconsistent data across time and location might reduce prediction accuracy.
2. Model Complexity
- Integration of Diverse Data: Combining data types such as weather, soil, and crop information necessitates advanced algorithms and processing resources.
- Complex interconnections: It is difficult to capture the intricate interconnections of biological, physical, and chemical processes in agricultural systems.
3. Adaptation to Local Conditions:
- Regional Specificity: To be effective, models must be adapted to a region’s specific climatic, soil, and agricultural variables, which necessitates local calibration and validation.
- Scalability: Adapting models to different scales, such as small farms or huge agricultural districts, can be challenging.
4. Technology Access:
- Infrastructure: Farmers in developing nations may lack access to essential technology infrastructure, such as computers and internet access.
- Training and Expertise: Effective application of agro-meteorological models necessitates training and expertise, which may not be accessible to all farmers.
5. Climate Change Uncertainty:
- Unpredictable Weather Patterns: Climate change causes increasing variability and unpredictability in weather patterns, making precise forecasting more difficult.
- Long-term Projections: There are major uncertainty in predicting the long-term effects of climate change on agriculture.
6. Economic Constraints:
- Funding: Creating, maintaining, and upgrading agro-meteorological models can be expensive, necessitating a consistent financial investment.
- Affordability for Farmers: Small-scale farmers may find it prohibitively expensive to obtain and apply these approaches.
7. Interdisciplinary Collaboration:
- Coordination: Effective agro-meteorological modeling necessitates coordination among meteorologists, agronomists, soil scientists, and other professionals, which can be difficult to achieve.
- Communication: Ensuring that model outputs are adequately communicated to farmers and stakeholders in an understandable format.
Agro-meteorological models are valuable tools for optimizing agricultural practices by combining weather, soil, crop, and geographic data. They help farmers make informed decisions about irrigation, planting, harvesting, and pest control by providing accurate weather predictions, climate projections, and resource management information. While these models greatly improve agricultural productivity and sustainability, they confront several obstacles, including data quality, model complexity, adaptation to local conditions, and accessibility issues, particularly in developing countries. Overcoming these issues is critical for realizing their full promise in creating efficient and resilient agricultural systems.
Frequently Asked Question(FAQ)
What are agro-meteorological models?
Agro-meteorological models use weather, soil, crop, and geographic data to enhance agricultural methods. They offer insights and projections for irrigation, planting, harvesting, and pest management, which improve productivity and sustainability.
What are some examples of agro-meteorological models?
Examples include DSSAT (Decision Support System for Agrotechnology Transfer) and APSIM (Agricultural Production Systems Simulator) for crop growth, WRF (Weather Research and Forecasting) for weather forecasting, and EPIRICE for rice pest and disease prediction.
Related Articles